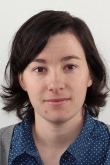
January 10, 2014
Carmela Troncoso
In this talk we explore the suitability of Bayesian Inference techniques, specifically Markov Chain Monte Carlo methods, to evaluate information leakage in complex scenarios. Using anonymity systems, in particular mix networks, as case study we show that casting problems in the context of Bayesian inference provides an appropriate framework to evaluate security properties (e.g., traceability of messages) in presence of complex constraints. We present a generative probabilistic model of mix network architectures that incorporates a number of attack techniques in the literature that exploit different constrants. We use the model to build a Markov Chain Monte Carlo inference engine based on the Metropolis-Hastings algorithm that calculates the probabilities of who is talking to whom given an observation of the anonymity network. Finally, we briefly overview other Bayesian techniques useful to tackle other security problems, such as Gibbs sampling suitable for user profiling, or particle filtering that allows to consider dynamic behaviour.