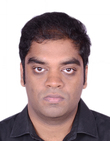
February 21, 2018
Deepak Padmanabhan
Conventional unsupervised data analytics techniques have largely focused on processing datasets of single-type data, e.g., one of text, ECG, Sensor Readings and Image data. With increasing digitization, it has become common to have data objects having representations that encompass different “kinds” of information. For example, the same disease condition may be identified through EEG or fMRI data. Thus, a dataset of EEG-fMRI pairs would be considered as a parallel two-view dataset. Datasets of text-image pairs (e.g., a description of a seashore, and an image of it) and text-text pairs (e.g., problem-solution text, or multi-language text from machine translation scenarios) are other common instances of multi-view data. The challenge in multi-view exploratory analytics is about effectively leveraging such parallel multi-view data to perform analytics tasks such as clustering, retrieval and anomaly detection. This talk will cover some emerging trends in processing multi-view parallel data and outline the speaker’s research plan in the area. This talk will cover two recent research publications authored by the speaker, one on multi-view clustering, and another on multi-view dimensionality reduction.